Reuben College has welcomed its first ten Schmidt AI in Science Fellows
In October 2022, the University of Oxford became one of nine leading research universities around the world selected to deliver a new global postdoctoral fellowship programme to drive the innovative use of artificial intelligence within science, technology, engineering and mathematics (STEM) research.
The Eric and Wendy Schmidt AI in Science Postdoctoral Fellowship, a programme of Schmidt Futures, aims to accelerate the next scientific revolution by supporting talented postdoctoral researchers to apply AI techniques to research problems in the natural sciences, engineering and mathematical sciences.
The programme is entirely cross-disciplinary, spanning the breadth of the Mathematical, Physical and Life Sciences (MPLS) Division, and fosters an environment of building collaborations, by focussing on bringing together different parts of the AI landscape in innovative and novel ways.
The Eric and Wendy Schmidt AI in Science Fellows are also Associate Research Fellows at Reuben College. They were welcomed to the University at a joint event with Reuben on Thursday 4 May 2023, during which they each outlined the focus of their research. They then went on to a talk and dinner at the University Museum of Natural History, experiencing ‘Dining with Dinosaurs’ for the first time.
The College hopes to welcome another 20 Schmidt Associate Research Fellows to the college this October, and is looking forward to the contribution they will make to the Reuben community while they are with us.
You can find out more about each of the Fellows below, and read the full press release and details of the programme on the MPLS website.
Extracting useful knowledge from the aggregated load at substations level plays an important role in the planning and operation of the distribution grid – enabling smart solutions for demand-side energy management as well as fault detection and recovery. The research aims to develop a learning-based method to extract information from existing grid measurements (active and reactive power, voltage and current).
The project aims to develop an AI to automatically calibrate the changes in device parameters of a quantum processor, to maintain its stable performance. This will significantly benefit both academia and industry for controlling large-scale superconducting quantum processors and has the potential to be adapted to other types of quantum processors.
Richard Creswell - “Applications of AI/ML to epidemiological time series”
Infectious diseases such as COVID-19 represent an important target for mathematical models which describe how a disease progresses through a population. A model’s parameter values may be informed by prior knowledge of the disease, but often they must be inferred from data such as the daily number of deaths or weekly number of positive tests. This raises numerous challenges which will benefit from the application of advanced techniques from AI and ML. The lessons learned are expected to apply directly to other problems.
Qi Hu - “Embedded AI for next generation biomedical optical imaging systems”
Optical microscopes suffer from distortions introduced by imperfect hardware and innate sample structures that can detrimentally affect image quality. Adaptive optics (AO) uses reconfigurable devices to correct aberrations, but such methods have limitations. The work will embed neural network algorithms into the feedback control of the AO system and lead to improvements in performance.
Holly Pacey - “Maximising LHC discovery potential with graph neural networks (GNNs)”
The LHC's ATLAS experiment collides protons at near-light speeds, with the goal of discovering evidence for beyond Standard Model (BSM) particles. However, BSM searches to date use only properties of each individual collision to discriminate between BSM- and SM-like collisions. The project will use graph neural networks (GNN) analysis to revolutionise ‘anomaly detection’.
Rachel Parkinson - “Employing AI to identify the complex interactions of environmental stressors on pollinator health”
There is a critical need to investigate how environmental change affects pollinator behaviour so that steps can be taken to mitigate economic and ecological risk. Sound is a typically overlooked component of behaviour, and the project will result in a powerful tool for integrating computer vision and sound to automatically track the behaviour of insects. The technology will have application in the risk assessment of environmental stressors.
Carlos Outeiral Rubiera - “Protein expression optimization using large language models”
Engineered proteins, a crucial ingredient in vaccines, medicines, and diagnostic tests, pose production challenges due to the complex DNA 'dialects' used by protein-producing microbes. Even small dialectal mismatches in the engineered DNA can impair the metabolic machinery and greatly reduce yield. Our project will use AI to 'translate' between DNA dialects to enhance protein production, aiming to show the potential of artificial intelligence in synthetic biology.
Heloise Stevance - “A Virtual Research Assistant for the next generation of sky surveys”
Starting in 2024, the Rubin Observatory will record the most exhaustive sky-survey humanity has ever undertaken: it will detect the explosions of stars hundreds of millions of light-years away, seeing 10 million new events each night. The project will create a model that is intelligent enough to serve as a virtual research assistant and allow human scientists to fully exploit this revolutionary source of astrophysical data.
Tianning (Tim) Tang - “Intelligent wave breaking characterisation with machine learning”
The project will use machine learning (ML) to characterise the initiation of wave breaking and seek a novel equation-based description of the wave breaking discovered by ML. The ultimate aim is to discover the formulation describing the breaking process after the initiation. This would have benefits in ocean engineering but also contribute to the ultimate question: can we discover physics with ML?
Jake Taylor - “Modelling Exoplanet Atmospheres with Machine Learning”
Modelling the atmospheres of exoplanets is a computationally demanding task and becoming more demanding in the James Webb Space Telescope era. The project will use machine learning to emulate the calculation of molecular opacity. Combining this new tool with GPU programming will significantly improve our ability to interpret the atmospheres of these alien worlds.
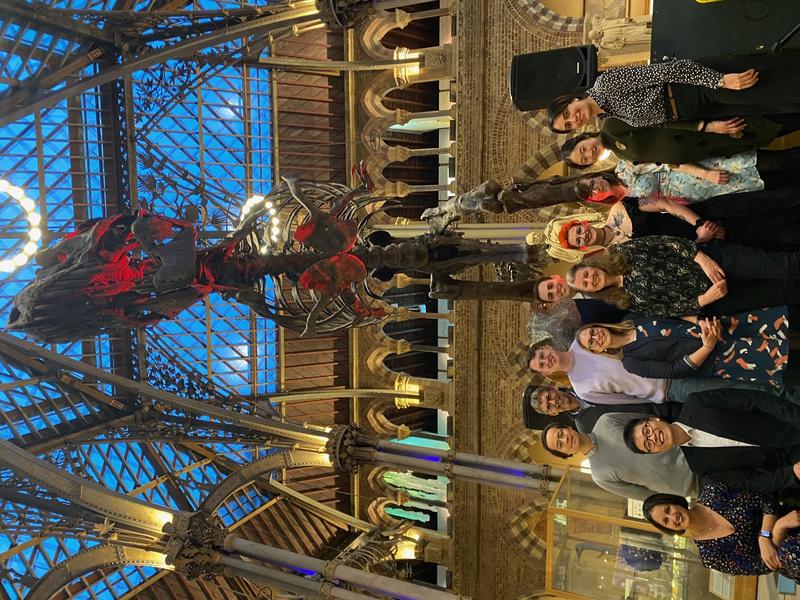